Learning and Using the Arrow of Time
4. Massachusessets Institute of Technology 5. Google Research
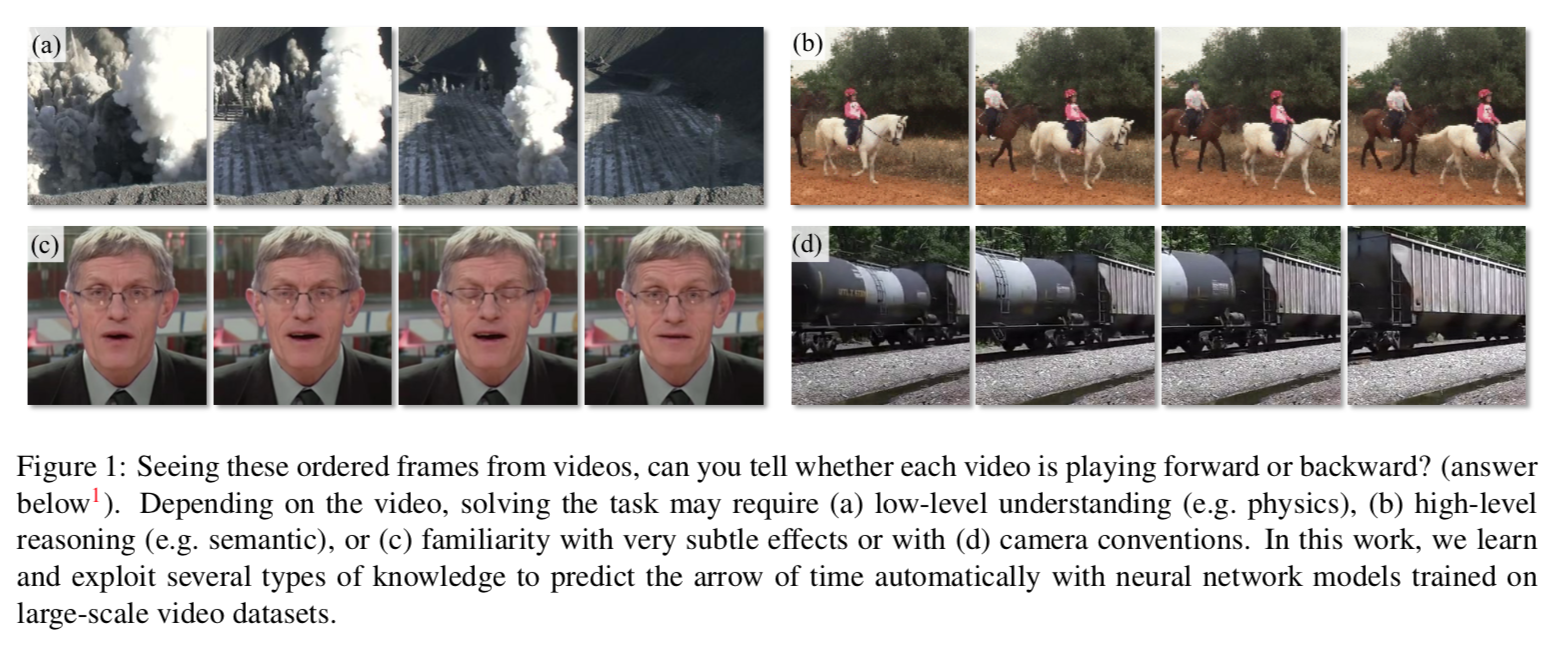
Abstract
We seek to understand the arrow of time in videos--what makes videos look like playing forwards or backwards? Can we visualize the cues? Can the arrow of time be a supervisory signal useful for activity analysis? To this end, we apply a learning-based approach to a large set of videos.
To learn the arrow of time efficiently and reliably, we design a ConvNet suitable for extended temporal footprints and for the class activation visualization, and study the effect of artificial cues, such as inematographic conventions, on learning. Our trained model achieves the state-of-the-art performance on two large-scale real-world video datasets. Through cluster analysis, we examine the learned visual cues, showing when and where they occur. Lastly, we use the trained ConvNet for two applications: self-supervision for action recognition, and video forensics -- determining whether Hollywood film clips have been deliberately reversed in time as special effects.
Paper Video
Citation
Bibilographic information for this work:
@inproceedings{wei2018learning, title={Learning and Using the Arrow of Time}, author={Wei, Donglai and Lim, Joseph J and Zisserman, Andrew and Freeman, William T}, booktitle={Proceedings of the IEEE Conference on Computer Vision and Pattern Recognition}, pages={8052--8060}, year={2018} }
Acknowledgement
This work was supported by NSF Grant 1212849 (Reconstructive Recognition), and by the EPSRC Programme Grant Seebibyte EP/M013774/1